17. Diffusive Response#
Note
The Python scripts used below and some materials are modified from Prof. Brian E. J. Rose’s climlab website.
%matplotlib inline
import numpy as np
import matplotlib.pyplot as plt
import climlab
# for convenience, set up a dictionary with our reference parameters
param = {'A':210, 'B':2, 'a0':0.3, 'a2':0.078, 'ai':0.62, 'Tf':-10.}
model1 = climlab.EBM_annual(name='Annual EBM with ice line',
num_lat=180, D=0.55, **param )
print( model1)
print(model1.param)
def ebm_plot( model, figsize=(8,12), show=True ):
'''This function makes a plot of the current state of the model,
including temperature, energy budget, and heat transport.'''
templimits = -30,35
radlimits = -340, 340
htlimits = -7,7
latlimits = -90,90
lat_ticks = np.arange(-90,90,30)
fig = plt.figure(figsize=figsize)
ax1 = fig.add_subplot(3,1,1)
ax1.plot(model.lat, model.Ts)
ax1.set_xlim(latlimits)
ax1.set_ylim(templimits)
ax1.set_ylabel('Temperature (°C)')
ax1.set_xticks( lat_ticks )
ax1.grid()
ax2 = fig.add_subplot(3,1,2)
ax2.plot(model.lat, model.ASR, 'k--', label='SW' )
ax2.plot(model.lat, -model.OLR, 'r--', label='LW' )
ax2.plot(model.lat, model.net_radiation, 'c-', label='net rad' )
ax2.plot(model.lat, model.heat_transport_convergence, 'g--', label='dyn' )
ax2.plot(model.lat, model.net_radiation
+ model.heat_transport_convergence, 'b-', label='total' )
ax2.set_xlim(latlimits)
ax2.set_ylim(radlimits)
ax2.set_ylabel('Energy budget (W m$^{-2}$)')
ax2.set_xticks( lat_ticks )
ax2.grid()
ax2.legend()
ax3 = fig.add_subplot(3,1,3)
ax3.plot(model.lat_bounds, model.heat_transport)
ax3.set_xlim(latlimits)
ax3.set_ylim(htlimits)
ax3.set_ylabel('Heat transport (PW)')
ax3.set_xlabel('Latitude')
ax3.set_xticks( lat_ticks )
ax3.grid()
return fig
model1.integrate_years(5)
f = ebm_plot(model1)
model1.icelat
climlab Process of type <class 'climlab.model.ebm.EBM_annual'>.
State variables and domain shapes:
Ts: (180, 1)
The subprocess tree:
Annual EBM with ice line: <class 'climlab.model.ebm.EBM_annual'>
LW: <class 'climlab.radiation.aplusbt.AplusBT'>
insolation: <class 'climlab.radiation.insolation.AnnualMeanInsolation'>
albedo: <class 'climlab.surface.albedo.StepFunctionAlbedo'>
iceline: <class 'climlab.surface.albedo.Iceline'>
warm_albedo: <class 'climlab.surface.albedo.P2Albedo'>
cold_albedo: <class 'climlab.surface.albedo.ConstantAlbedo'>
SW: <class 'climlab.radiation.absorbed_shorwave.SimpleAbsorbedShortwave'>
diffusion: <class 'climlab.dynamics.meridional_heat_diffusion.MeridionalHeatDiffusion'>
{'timestep': 350632.51200000005, 'S0': 1365.2, 's2': -0.48, 'A': 210, 'B': 2, 'D': 0.55, 'Tf': -10.0, 'water_depth': 10.0, 'a0': 0.3, 'a2': 0.078, 'ai': 0.62}
Integrating for 450 steps, 1826.2110000000002 days, or 5 years.
Total elapsed time is 5.000000000000044 years.
array([-70., 70.])
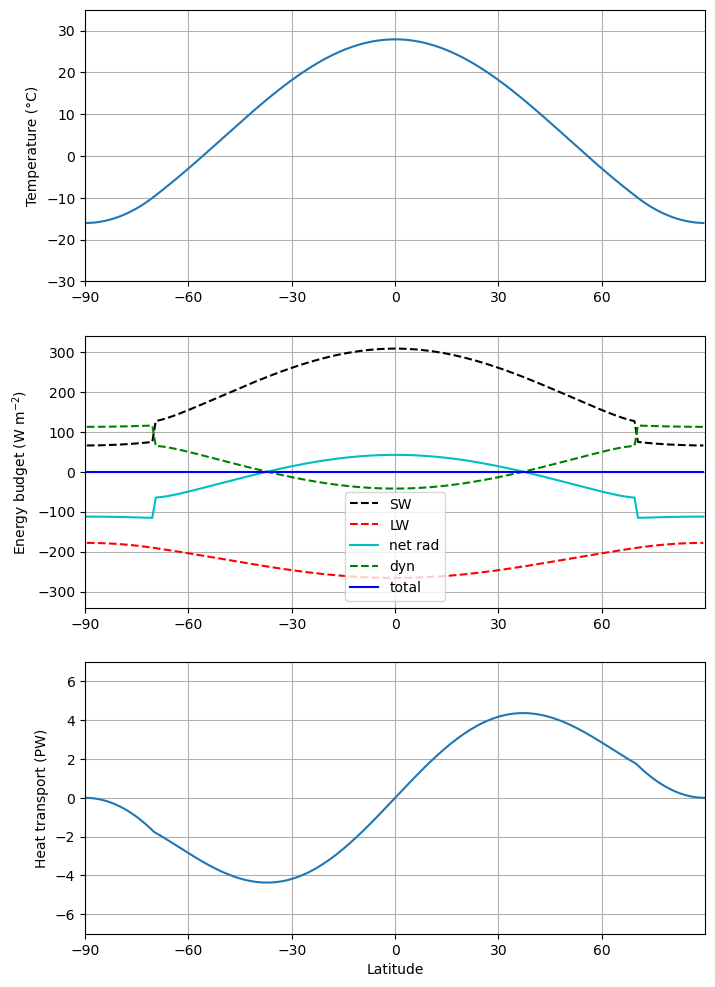
deltaA = 4.
model2 = climlab.process_like(model1)
model2.subprocess['LW'].A = param['A'] - deltaA
model2.integrate_years(5, verbose=False)
plt.plot(model1.lat, model1.Ts)
plt.plot(model2.lat, model2.Ts)
model2.icelat
array([-90., 90.])
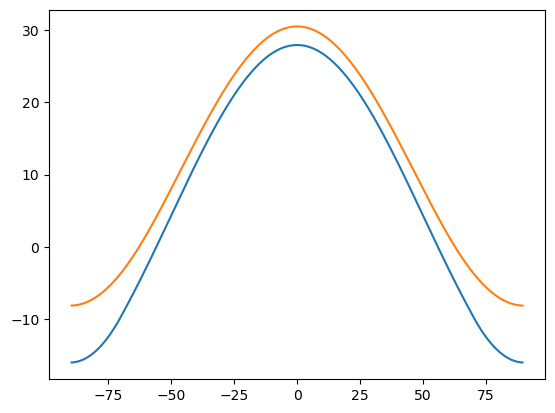
model3 = climlab.process_like(model1)
model3.subprocess['LW'].A = param['A'] - 2*deltaA
model3.integrate_years(5, verbose=False)
plt.plot(model1.lat, model1.Ts)
plt.plot(model2.lat, model2.Ts)
plt.plot(model3.lat, model3.Ts)
plt.xlim(-90, 90)
plt.grid()
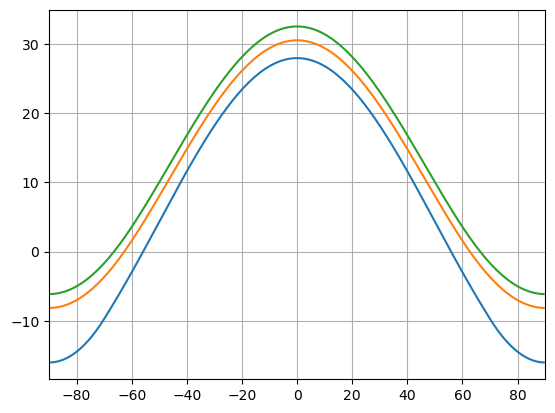
The effect of diffusivity with albedo feedback#
We repeat the results of Stone (1978)
param = {'A':210, 'B':2, 'a0':0.3, 'a2':0.078, 'ai':0.62, 'Tf':-10.}
print( param)
Darray = np.arange(0., 2.05, 0.05)
model_list = []
Tmean_list = []
deltaT_list = []
Hmax_list = []
for D in Darray:
ebm = climlab.EBM_annual(num_lat=180, D=D, **param )
ebm.integrate_years(5., verbose=False)
Tmean = ebm.global_mean_temperature()
deltaT = np.max(ebm.Ts) - np.min(ebm.Ts)
HT = np.squeeze(ebm.heat_transport)
ind = np.where(ebm.lat_bounds==35.)[0]
Hmax = HT[ind]
model_list.append(ebm)
Tmean_list.append(Tmean)
deltaT_list.append(deltaT)
Hmax_list.append(Hmax)
color1 = 'b'
color2 = 'r'
fig = plt.figure(figsize=(8,6))
ax1 = fig.add_subplot(111)
ax1.plot(Darray, deltaT_list, color=color1, label=r'$\Delta T$')
ax1.plot(Darray, Tmean_list, '--', color=color1, label=r'$\overline{T}$')
ax1.set_xlabel('D (W m$^{-2}$ °C$^{-1}$)', fontsize=14)
ax1.set_xticks(np.arange(Darray[0], Darray[-1], 0.2))
ax1.set_ylabel(r'Temperature (°C)', fontsize=14, color=color1)
for tl in ax1.get_yticklabels():
tl.set_color(color1)
ax1.legend(loc='center right')
ax2 = ax1.twinx()
ax2.plot(Darray, Hmax_list, color=color2)
ax2.set_ylabel('Poleward heat transport across 35.5° (PW)', fontsize=14, color=color2)
for tl in ax2.get_yticklabels():
tl.set_color(color2)
ax1.set_title('Effect of diffusivity on EBM with albedo feedback', fontsize=16)
ax1.grid()
{'A': 210, 'B': 2, 'a0': 0.3, 'a2': 0.078, 'ai': 0.62, 'Tf': -10.0}
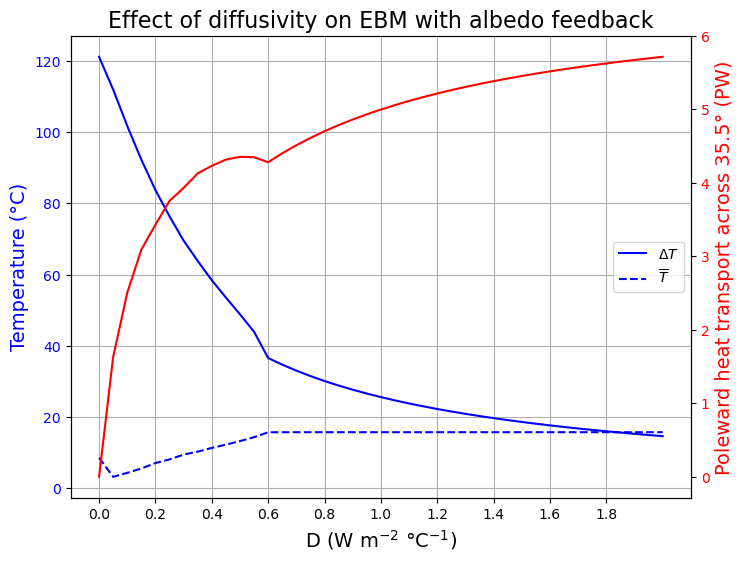
Diffusive response to a point source of energy at 45\(^o\)N#
without albedo feedback
param_noalb = {'A': 210, 'B': 2, 'D': 0.55, 'Tf': -10.0, 'a0': 0.3, 'a2': 0.078}
m1 = climlab.EBM_annual(num_lat=180, **param_noalb)
print(m1)
m1.integrate_years(5.)
m2 = climlab.process_like(m1)
point_source = climlab.process.energy_budget.ExternalEnergySource(state=m2.state, timestep=m2.timestep)
ind = np.where(m2.lat == 45.5)
point_source.heating_rate['Ts'][ind] = 100.
m2.add_subprocess('point source', point_source)
print( m2)
m2.integrate_years(5.)
plt.plot(m2.lat, m2.Ts - m1.Ts)
plt.xlim(-90,90)
plt.xlabel('Latitude')
plt.ylabel(r'$\Delta T_s$')
plt.grid()
climlab Process of type <class 'climlab.model.ebm.EBM_annual'>.
State variables and domain shapes:
Ts: (180, 1)
The subprocess tree:
Untitled: <class 'climlab.model.ebm.EBM_annual'>
LW: <class 'climlab.radiation.aplusbt.AplusBT'>
insolation: <class 'climlab.radiation.insolation.AnnualMeanInsolation'>
albedo: <class 'climlab.surface.albedo.P2Albedo'>
SW: <class 'climlab.radiation.absorbed_shorwave.SimpleAbsorbedShortwave'>
diffusion: <class 'climlab.dynamics.meridional_heat_diffusion.MeridionalHeatDiffusion'>
Integrating for 450 steps, 1826.2110000000002 days, or 5.0 years.
Total elapsed time is 5.000000000000044 years.
climlab Process of type <class 'climlab.model.ebm.EBM_annual'>.
State variables and domain shapes:
Ts: (180, 1)
The subprocess tree:
Untitled: <class 'climlab.model.ebm.EBM_annual'>
LW: <class 'climlab.radiation.aplusbt.AplusBT'>
insolation: <class 'climlab.radiation.insolation.AnnualMeanInsolation'>
albedo: <class 'climlab.surface.albedo.P2Albedo'>
SW: <class 'climlab.radiation.absorbed_shorwave.SimpleAbsorbedShortwave'>
diffusion: <class 'climlab.dynamics.meridional_heat_diffusion.MeridionalHeatDiffusion'>
point source: <class 'climlab.process.energy_budget.ExternalEnergySource'>
Integrating for 450 steps, 1826.2110000000002 days, or 5.0 years.
Total elapsed time is 9.999999999999863 years.
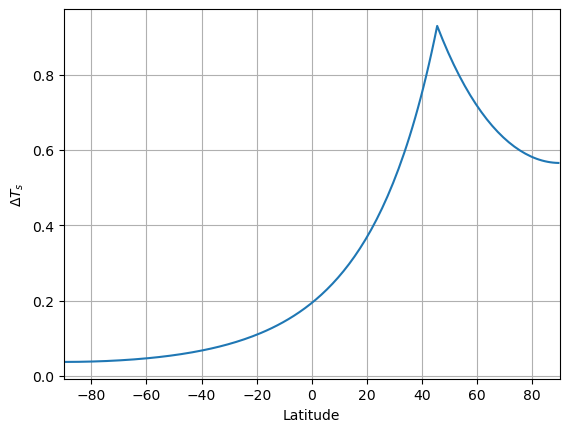
Note
The length scale of the point warming is suggested to be proportional to \(\sqrt{\frac{D}{B}}\)
with albedo
m3 = climlab.EBM_annual(num_lat=180, **param)
m3.integrate_years(5.)
m4 = climlab.process_like(m3)
point_source = climlab.process.energy_budget.ExternalEnergySource(state=m4.state, timestep=m4.timestep)
point_source.heating_rate['Ts'][ind] = 100.
m4.add_subprocess('point source', point_source)
m4.integrate_years(5.)
plt.plot(m4.lat, m4.Ts - m3.Ts)
plt.xlim(-90,90)
plt.xlabel('Latitude')
plt.ylabel(r'$\Delta T_s$')
plt.grid()
Integrating for 450 steps, 1826.2110000000002 days, or 5.0 years.
Total elapsed time is 5.000000000000044 years.
Integrating for 450 steps, 1826.2110000000002 days, or 5.0 years.
Total elapsed time is 9.999999999999863 years.
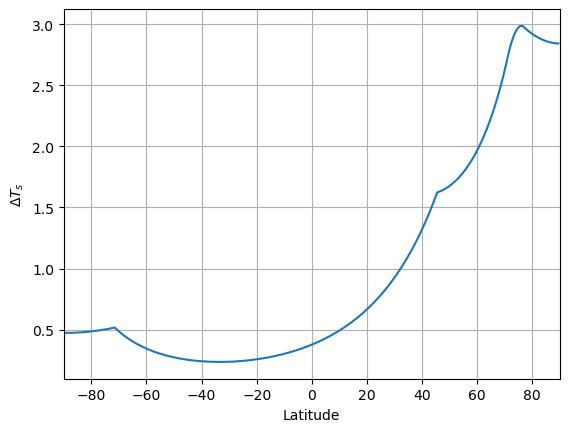